Research on Winter Jujube Object Detection Based on Optimized Yolov5s
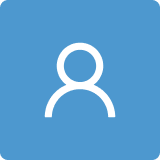
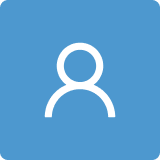
Round 1
Reviewer 1 Report
Manuscript info:
Journal: Agronomy MDPI
Title: Research on Winter Jujube Object Detection Based on 2 Optimized Yolov5s
Revision: --
Short summary
An improved Yolov5s algorithm was proposed in this study for the detection of winter jujube fruits in China. The improved model achieved a higher accuracy in detecting small and occluded fruits compared to the original Yolov5s algorithm by reducing the model size and network parameters, replacing the neck with a slim-neck, and optimizing the model with knowledge distillation. The optimized Yolov5s model outperformed the original Yolov5s in terms of precision, recall, mAP, and F1 values, while reducing the model size and parameters by 86.09% and 88.77%, respectively. This improved algorithm has the potential for real-time and accurate detection of small target fruits and can be applied to other jujube species. However, the optimized model had poor performance in backlighting scenes and this issue will be addressed in future work.
Strengths:
- The research paper is well-written and is relatively easy to read.
- Clear Problem Statement: The paper clearly states the problem of winter jujube target detection, which makes it easy for readers to understand the purpose of the research.
- Comparison of Different Algorithms: The paper compares the performance of different algorithms, including YOLOv3-tiny, YOLOv4-tiny, SSD, Faster RCNN and optimized YOLOv5s, which provides valuable information for readers who are interested in target detection.
- Evaluation Metrics: The paper uses several evaluation metrics, including precision, recall, mAP, and F1 score, which provides a comprehensive evaluation of the performance of different algorithms.
- Real-time Performance: The paper focuses on real-time detection, and the results show that the optimized YOLOv5s model has a fast FPS, which satisfies the requirement of real-time detection in embedded devices.
Weaknesses:
- Limited Generalizability: The research only focuses on winter jujube target detection and may not be applicable to other types of target detection.
- Lack of in-depth analysis: The paper only briefly discussed the results of the study, without a detailed analysis of the results and the limitations of the study. To resolve this, the researchers could provide more in-depth analysis and interpretation of the results in future studies.
- Backlighting Limitation: The paper mentions that the optimized model does not perform well in backlighting environments, which is a significant gap in the research.
In conclusion, the research paper provides valuable information about the performance comparison of different algorithms for winter jujube target detection. However, the limited generalizability and backlighting limitation should be addressed in future work.
Remarks:
v Why do you use Deep Learning? To me sounds not like a very difficult problem maybe even for a simple unsupervised clustering method. Would be good to include non-deep methods in the literature review, and explain why the problem is difficult (if it actually is)
v In section 2.3.3 (Slim-neck), the authors need to explain more about how the VoV-GSCSP model manages to simplify the computation and network structure while still preserving an acceptable level of accuracy.
v The description of Fig. 5 is not very clear. For better understanding, please add more descriptions of the image.
Author Response
Please see the attachment.
Author Response File: Author Response.pdf
Reviewer 2 Report
Author did not adress the occluded images and different lighting condition image. Again how IoU improve the detection process is not adress. Again the must important thing the dataset is not shared. Without knowing the imaging condition of images, its difficult to say anything about detection model specially in acse of natural images.
Author Response
Please see the attachment.
Author Response File: Author Response.pdf