Predicting Dissolution Kinetics of Tricalcium Silicate Using Deep Learning and Analytical Models
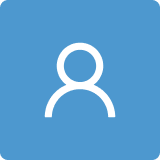
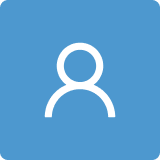
Round 1
Reviewer 1 Report
They want to understand the dissolution kinetics of Portland cement, key to controlling the hydration and optimizing the performance of concrete.
C3S … tricalcium silicate
The DF model is employed to predict the dissolution rate of C3S.
Apparently, the dissolution kinetics of C3S at early stages still remains a controversial subject.
The analytical models to describe the dissolution kinetics are also completely analytical because each model has constants which are fitted in it.
Ad: 99 – 101:
Fitting parameters in a model will always be accompanyied with errors be it human or numerical, as long as we don’t have any ab initio methods for dissolution kinetics, which we don’t.
Ad: 121:
Remarkable performance, in terms of R² ~ 0.98 could be also due to overfitting, please keep that in mind. Even if ML, DL, DF, NN, …. algorithms sound nice and fency at the end it is fitting a high dim function to a function with lover dimensions according to the Kolmogorov-Arnold representation Theorem:
Andrey Kolomogorv: "On the representation of continuous functions of several variables by superpositions of continuous functions of a smaller number of variables", Proceedings of the USSR Academy of Sciences, 108 (1956), pp. 179–182; English translation: Amer. Math. Soc. Transl., 17 (1961), pp. 369–373. Vladimir Arnold, "On functions of three variables", Proceedings of the USSR Academy of Sciences, 114 (1957), pp. 679–681; English translation: Amer. Math. Soc. Transl., 28 (1963), pp. 51–54.
Maybe you could emphasize this fact in this sentence.
Ad: 125-126:
Exactly no model has shown that ML provides a valid approach to predict such problems, which also includes this study!
Ad: 149: must be a typo concertation!
The authors have developed an analytical model with the help of the ML-DF method. If the model can be used in a wider range then the other models from table1 will be seen in the future. I can not see the big advantage of this model to the others, some models from table1 are definitely applicable to a wider range than the new model from the authors with the 7 (C1-C7) fitted constants in mind.
The generating of this analytical model with the aid of ML-DF seems to be a new idea and I think such an approach will be used by many other scientists in many other fields in the future.
Author Response
Please see the attached file
Author Response File: Author Response.pdf
Reviewer 2 Report
The goal of this paper is to develop (high-fidelity) models that predict the dissolution rate of C3S at the undersaturation state. The authors argue that the state-of-the-art analytical models cannot produce reliable predictions, which motivates this work. First, a deep forest (DF) model is trained on data sets generated by two dissolution measurements methods taken from two publications in the literature: (1) reactor connected to inductive coupled plasma spectrometer and (2) flow chamber with vertical scanning interferometry. The authors argue that the trained models show good predictive performance on independent test data sets. Based on the feature importance ranking from the DF model, the authors extend the Lasaga et al model to develop an analytical expression for C3S dissolution rate. The authors use the data of Nicoleau et al (that uses the reactor connected to inductive coupled plasma spectrometer) to independently validate the analytical model based on generic and alkaline solvents.
Overall, this is an interesting work. Leveraging DF results to build analytical models that incorporate mechanistic insights is much appreciated, novel, and interesting. I have several clarifying questions for the authors to consider before this manuscript can be recommended for publication.
1. Why is undersaturation state very crucial to develop a quantitative understanding of the C3S dissolution? Can the authors provide a more clear rationale for this regime with relevant citations? Are near-saturation state and undersaturation state synonymous?
2. Some of the terms in Table 1 are not defined or have typos (e.g., n1 and n2), which is distracting.
3. What is the definition of the term "high-fidelity" in this paper according to the authors? Does it mean R^2 > 0.9? In the ML literature, high-fidelity typically refers to first-principles based mechanistic models that are computationally expensive but capture the physics of the problem. I think the authors' definition of high-fidelity is quite different, as a result it must be stated clearly to avoid confusion.
4. The authors state that "This study only investigates the dissolution kinetics during the initial period." What is the connection between this sentence and the near-saturation or undersaturation state? Can they discuss this in the paper?
5. The authors state that "... the DF models can proficiently learn the cause-effect correlation ..." This is problematic because if one is exploring cause-effect relationship that several underlying assumptions/conditions must be stated (see the papers of Judea Pearl and colleagues). I strongly recommend the authors to remove the "cause-effect" language from the paper or justify it more clearly. In my opinion, the DF model is exploiting the correlation structure to build input-output relationships.
6. In section 4.0, the authors state that "outliers should be included in the database to ensure diversity." In my opinion, under the i.i.d. assumption in statistical learning theory outliers are unlikely. If they are present then they must be identified and removed. Did the authors find outliers in the data considered in this paper? What constitutes an outlier in this paper? Can they discuss in detail? How did the presence/absence of outliers impact their model performance?
7. In section 4.0, the authors state that "The DF model exhibits much better performance than analytical models, ..." I do not see any comparison anywhere. What is the rationale for this statement? Where are the results?
8. What is the origin of Equation 1 (in line 278)? or How did they arrive at this equation? I see no citation in the paper. Can this be clarified?
9. The authors argue that the moderate R^2 of 0.69 for the analytical model (compared to the DF model) was because it cannot account for all influential factors. What influential factors are missing in the analytical model? Adding a sentence or two would help because it will help the community to further improve the developed model.
Given these concerns, I am unable to recommend this version of the manuscript for publication.
Author Response
Please see the attached file.
Author Response File: Author Response.pdf
Round 2
Reviewer 1 Report
All my points have been adressed and the paper is ready to publish.
Author Response
The authors would like to thank the reviewer for their review and their consideration of this paper.
Reviewer 2 Report
I appreciate the detailed response from the authors. I believe the revised manuscript is much improved now. I have only one minor point for the authors to consider based on their revisions. This is about the "outliers". I really appreciate the authors for providing a clarification about the outliers, but I am unable to follow this stated logic: "Second, outliers should be included in the database to ensure that the DF model comprehensively learn input-output relationships". Do the authors have citations or empirical data (or better, any theoretical evidence) that demonstrate the validity of this statement?
Other than that I am happy to recommend this paper for publication.
Author Response
The authors would like to thank the reviewer for their review and their consideration of this paper. The authors have addressed all specific comments made by the reviewer. All corresponding changes in the manuscript are highlighted in yellow.
As we stated, outliers in this study defined as data-records that do not fit into the trends exhibited by majority of the data-records in the neighborhood because of some underlying (chemical, or kinetic, or thermodynamic) mechanism although measured and reported properly. These outliers may appear again in similar material systems and repeated experiments, and it is important to include them in the database to maintain the generalizability of the model. Chakravarty et al. (https://0-doi-org.brum.beds.ac.uk/10.1016/j.asoc.2020.106535) and Carlini et al. ( https://0-doi-org.brum.beds.ac.uk/10.48550/arXiv.1910.13427) have shown that the inclusion of outliers can ensure that the model captures all input-output correlations and improve prediction accuracy. The citations are added to the manuscript.