Information Theory and Information Geometry in Dynamical Systems and Machine Learning
A special issue of Entropy (ISSN 1099-4300). This special issue belongs to the section "Information Theory, Probability and Statistics".
Deadline for manuscript submissions: closed (30 December 2022) | Viewed by 3132
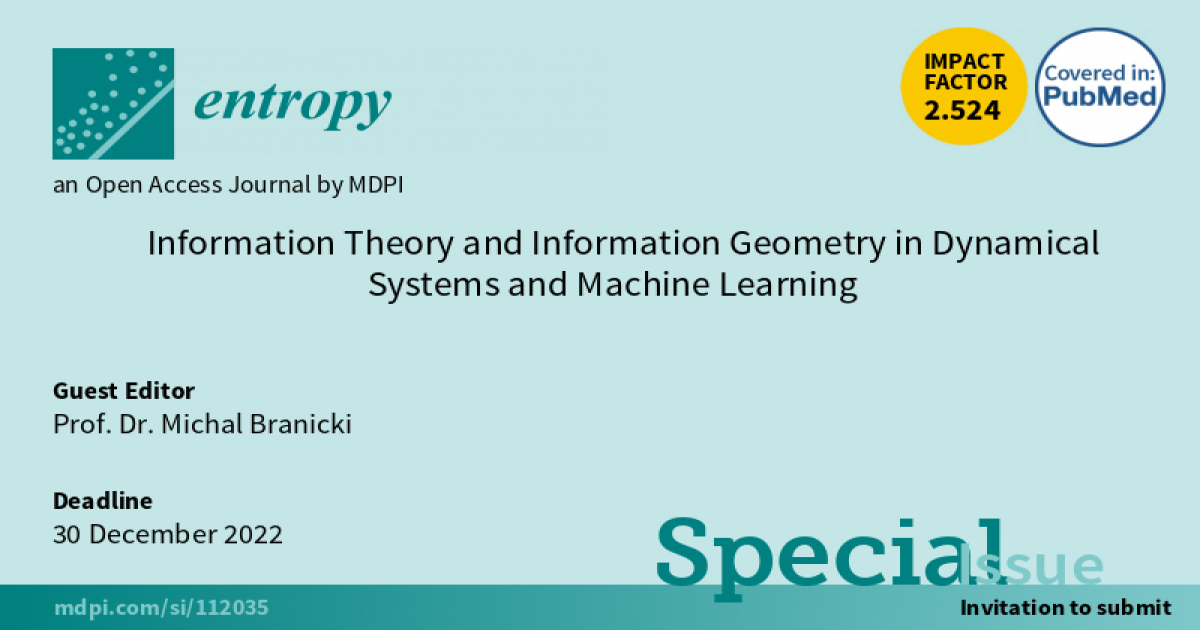
Special Issue Editor
2. The Alan Turing Institute for Data Science, London, UK
Interests: information theory and stochastics for uncertainty quantification in prediction of partially observed dynamical systems; stochastic filtering and data assimilation in high-dimensional dynamical systems; information geometry and mathemamtical foundations of machine learning
Special Issues, Collections and Topics in MDPI journals
Special Issue Information
Dear Colleagues,
Information theory and the evolution of its entropic functionals have long been known to be crucial for understanding important properties of dynamical systems, and for providing deep links to ergodic theory and statistical physics. The analysis of dynamical systems through the prism of information-bearing processes and symbolic dynamics enabled a rigorous description of entropic complexity and information content of underlying dynamics. The flow of information between components of a dynamical system allows one to study its predictability and the dynamic uncertainty, while quantifying the loss of information provides important tools for constructing coarse-grained approximations.
Information geometry and its inherent links to information theory on manifolds of probability measures has gained importance in the systematic analysis of statistical estimation, time series analysis, machine learning, or signal processing and optimization. Importantly, a number of these themes can be also approached through the theory of dynamical systems.
This Special Issue aims to serve as a timely locus for bringing together modern theoretical and application-oriented developments arising from the cross-fertilisation between information theory, information geometry, and dynamical systems theory. Contemporary applications to the quantification of predictability and dynamic uncertainty, as well as various aspects of learning theory, robustness, and approximation capacity of neural networks and other information processing systems, are of particular interest.
Dr. Michal Branicki
Guest Editor
Manuscript Submission Information
Manuscripts should be submitted online at www.mdpi.com by registering and logging in to this website. Once you are registered, click here to go to the submission form. Manuscripts can be submitted until the deadline. All submissions that pass pre-check are peer-reviewed. Accepted papers will be published continuously in the journal (as soon as accepted) and will be listed together on the special issue website. Research articles, review articles as well as short communications are invited. For planned papers, a title and short abstract (about 100 words) can be sent to the Editorial Office for announcement on this website.
Submitted manuscripts should not have been published previously, nor be under consideration for publication elsewhere (except conference proceedings papers). All manuscripts are thoroughly refereed through a single-blind peer-review process. A guide for authors and other relevant information for submission of manuscripts is available on the Instructions for Authors page. Entropy is an international peer-reviewed open access monthly journal published by MDPI.
Please visit the Instructions for Authors page before submitting a manuscript. The Article Processing Charge (APC) for publication in this open access journal is 2600 CHF (Swiss Francs). Submitted papers should be well formatted and use good English. Authors may use MDPI's English editing service prior to publication or during author revisions.
Keywords
- information theory
- information geometry
- dynamical systems
- ergodic theory
- machine learning
- neural networks
- Bayesian estimation
- data-driven models