Applications of Artificial Intelligence and Machine Learning in Wireless Communications and Networks
A special issue of Applied Sciences (ISSN 2076-3417). This special issue belongs to the section "Electrical, Electronics and Communications Engineering".
Deadline for manuscript submissions: closed (31 October 2022) | Viewed by 11544
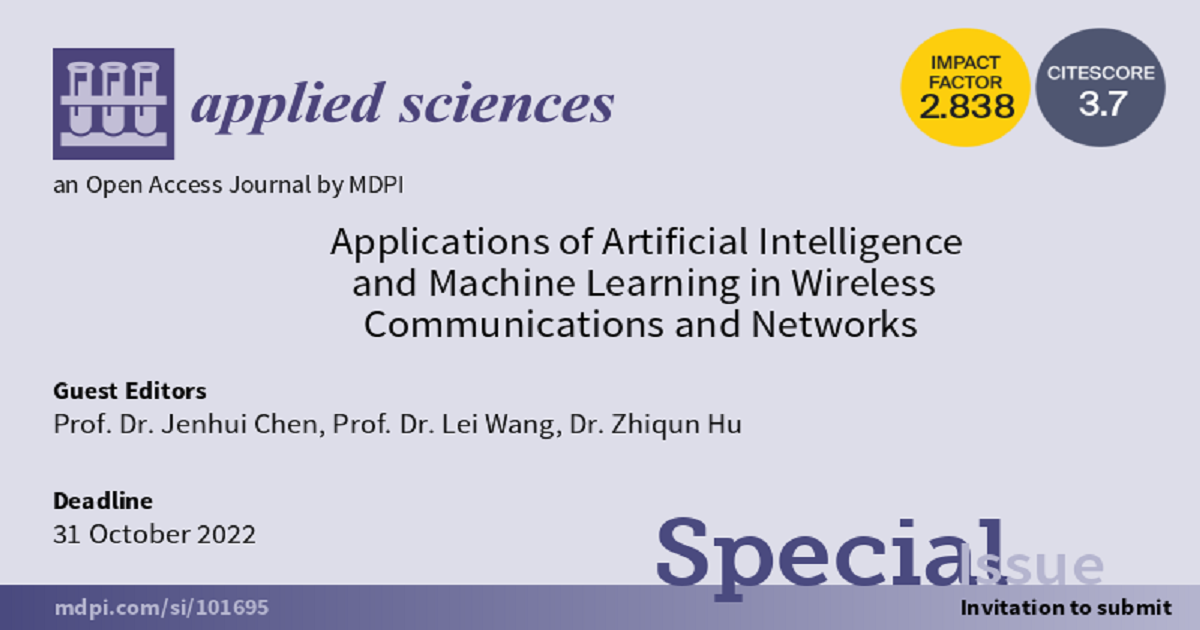
Special Issue Editors
Interests: multimedia; computer vision; wireless networks; stock prediction; natural language processing; Internet of Things
Special Issues, Collections and Topics in MDPI journals
Interests: wireless networking; AI; underwater robot
Special Issue Information
Dear Colleagues,
The great success of artificial intelligence (AI) and machine learning (ML) technologies has led to the possibility of developing more advanced communications and networks technologies. Deep neural network-based machine learning is a promising tool to overcome the greatest challenges in wireless communications and networks imposed by the increasing demands for improved capacity, coverage, latency, efficiency, flexibility, compatibility, and quality of experience. This Special Issue is dedicated to documenting the latest applications and results of AI and ML technologies in wireless communications and networks. We welcome articles on the following relevant topics:
- ML-based resource management;
- ML in energy efficiency optimization;
- ML in medium access control layer;
- Machine learning based quality of service (QoS) management in wireless networks;
- ML-based intelligent computing algorithms for wireless networks;
- Novel reinforcement learning (RL) methods for wireless networks;
- AI/ML for 5G and beyond;
- ML-Aided unmanned aerial vehicles (UAV) control;
- ML-based killer applications in future wireless communications and networks.
Submissions on other topics that are in accordance with the theme of the Special Issue are also welcome and may take the form of original research articles, review articles, or opinions on methodologies or applications.
Prof. Dr. Jenhui Chen
Prof. Dr. Lei Wang
Dr. Zhiqun Hu
Guest Editors
Manuscript Submission Information
Manuscripts should be submitted online at www.mdpi.com by registering and logging in to this website. Once you are registered, click here to go to the submission form. Manuscripts can be submitted until the deadline. All submissions that pass pre-check are peer-reviewed. Accepted papers will be published continuously in the journal (as soon as accepted) and will be listed together on the special issue website. Research articles, review articles as well as short communications are invited. For planned papers, a title and short abstract (about 100 words) can be sent to the Editorial Office for announcement on this website.
Submitted manuscripts should not have been published previously, nor be under consideration for publication elsewhere (except conference proceedings papers). All manuscripts are thoroughly refereed through a single-blind peer-review process. A guide for authors and other relevant information for submission of manuscripts is available on the Instructions for Authors page. Applied Sciences is an international peer-reviewed open access semimonthly journal published by MDPI.
Please visit the Instructions for Authors page before submitting a manuscript. The Article Processing Charge (APC) for publication in this open access journal is 2400 CHF (Swiss Francs). Submitted papers should be well formatted and use good English. Authors may use MDPI's English editing service prior to publication or during author revisions.
Keywords
- machine learning
- deep learning
- ML-based resource management
- ML-based networking
- mobility management
- transmission intelligence
- distributed intelligence
- AIoT
- ML-based killer applications