Deep Learning with Differential Equations
A special issue of Applied Sciences (ISSN 2076-3417). This special issue belongs to the section "Computing and Artificial Intelligence".
Deadline for manuscript submissions: closed (31 August 2022) | Viewed by 2571
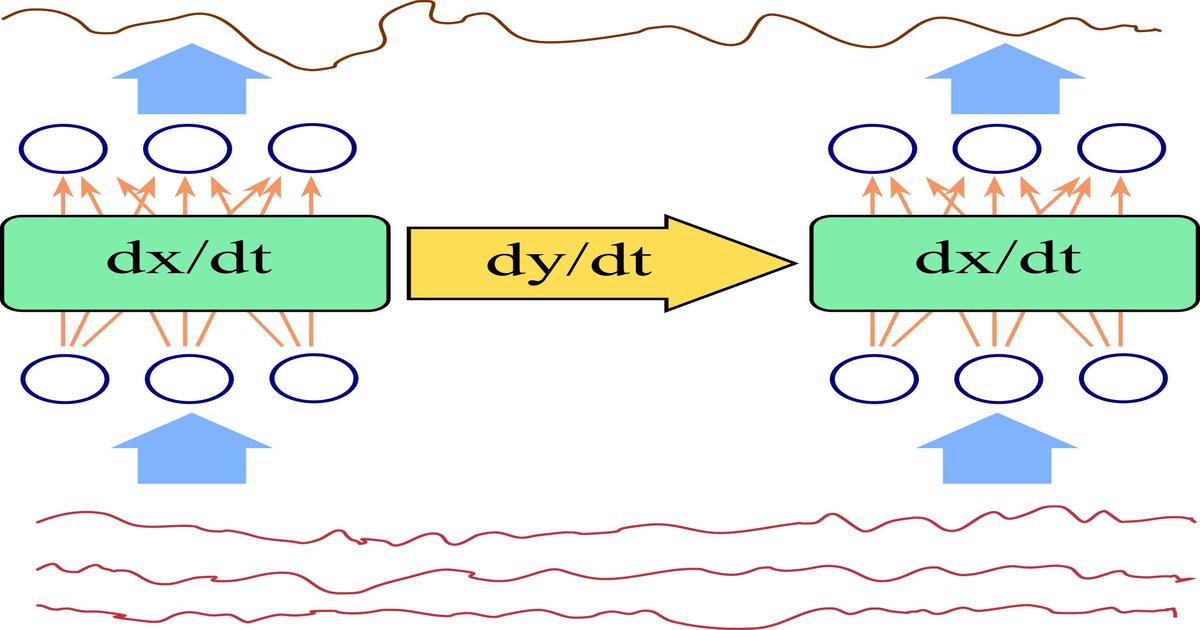
Special Issue Editor
Interests: Bayesian deep learning; neural stochastic processes; uncertainty quantification
Special Issues, Collections and Topics in MDPI journals
Special Issue Information
Dear Colleagues,
As artificial intelligence makes progress, deep neural nets are being applied to increasingly complex problem setups. In response to the emerging difficulties of these new setups, deep learning research explores new modeling tools to enhance the predictive power of neural nets. Differential equations are among the new tools that are being incorporated into deep neural net models in various ways. While some approaches use differential equations to build continuous depth into feed-forward neural networks, some others use them to induce a desired regularity or a conservation law into the dynamical system under investigation.
This Special Issue publishes original algorithmic, methodological, and theoretical contributions to artificial intelligence research regarding the incorporation of differential equations into the design of deterministic deep neural networks or the inference of probabilistic deep neural networks. The simplicity of a contribution is a value for us, providing that its significance is demonstrated in rigorous experiments. Theoretical analyses of new aspects of existing approaches and justifications of new approaches using existing theoretical tools are very welcome.
This Special Issue targets a readership from multiple disciplines, including but not limited to machine learning, computer vision, robotics, bioinformatics, as well as business analysis and computational finance.
We look forward to your submissions!
Best regards,
Prof. Dr. Melih Kandemir
Guest Editor
Manuscript Submission Information
Manuscripts should be submitted online at www.mdpi.com by registering and logging in to this website. Once you are registered, click here to go to the submission form. Manuscripts can be submitted until the deadline. All submissions that pass pre-check are peer-reviewed. Accepted papers will be published continuously in the journal (as soon as accepted) and will be listed together on the special issue website. Research articles, review articles as well as short communications are invited. For planned papers, a title and short abstract (about 100 words) can be sent to the Editorial Office for announcement on this website.
Submitted manuscripts should not have been published previously, nor be under consideration for publication elsewhere (except conference proceedings papers). All manuscripts are thoroughly refereed through a single-blind peer-review process. A guide for authors and other relevant information for submission of manuscripts is available on the Instructions for Authors page. Applied Sciences is an international peer-reviewed open access semimonthly journal published by MDPI.
Please visit the Instructions for Authors page before submitting a manuscript. The Article Processing Charge (APC) for publication in this open access journal is 2400 CHF (Swiss Francs). Submitted papers should be well formatted and use good English. Authors may use MDPI's English editing service prior to publication or during author revisions.
Keywords
- Neural Ordinary Differential Equations
- Neural Stochastic Differential Equations
- Neural Processes
- Normalizing Flows
- Physics-Informed Neural Networks
- Deep Equilibrium Models
- Hamiltonian Networks
- Latent Force Models
- Gaussian Processes
- Time Series Modeling
- Dynamics Modeling