Pattern Recognition in Multimedia Signal Analysis
A special issue of Applied Sciences (ISSN 2076-3417). This special issue belongs to the section "Computing and Artificial Intelligence".
Deadline for manuscript submissions: closed (30 April 2022) | Viewed by 30728
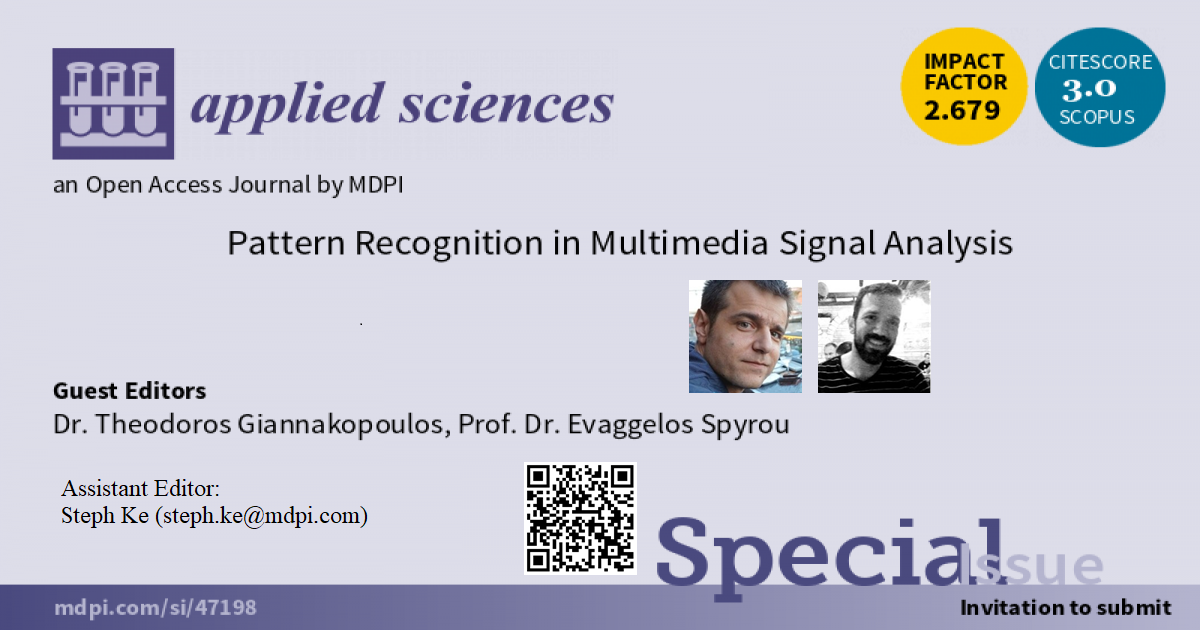
Special Issue Editors
Interests: audio/speech analysis; multimodal information retrieval
Interests: multimedia analysis; computer vision; human activity recognition; emotion recognition; deep learning
Special Issues, Collections and Topics in MDPI journals
Special Issue Information
Dear Colleagues,
Huge amounts of multimedia data have been generated in recent years, either through profesional “content providers” (TV, movies, internet TV, and music videos) or user-generated content (vlogs, social media multimodal content, and multisensor data). Therefore, the need for automatic indexing, classification, content visualization, and recommendation, through multimodal pattern recognition, is obvious for various applications. In addition, multimedia data exhibit much richer structures and representations than simple forms of data and, as a result, the related pattern recognition approaches must take that into consideration.
This Special Issue focuses on novel approaches for analyzing multimodal content using pattern recognition and signal analysis algorithms. Application areas include but are not limited to video summarization, content-based multimedia indexing and retrieval, content-based recommender systems, multimodal behavior and emotion recognition, patient/elderly home monitoring based on multimodal sensors, mental health monitoring, and autonomous driving.
In this Special Issue, we invite submissions that report on cutting-edge research in the broad spectrum of pattern recognition in multimedia analysis, related to the aforementioned areas. Survey papers and reviews in a specific research and/or application area are also welcome. All submitted papers will undergo our standard peer-review procedure. Accepted papers will be published in open-access format in Applied Sciences and collected together on this Special Issue website.
Dr. Theodoros Giannakopoulos
Prof. Dr. Evaggelos Spyrou
Guest Editor
Manuscript Submission Information
Manuscripts should be submitted online at www.mdpi.com by registering and logging in to this website. Once you are registered, click here to go to the submission form. Manuscripts can be submitted until the deadline. All submissions that pass pre-check are peer-reviewed. Accepted papers will be published continuously in the journal (as soon as accepted) and will be listed together on the special issue website. Research articles, review articles as well as short communications are invited. For planned papers, a title and short abstract (about 100 words) can be sent to the Editorial Office for announcement on this website.
Submitted manuscripts should not have been published previously, nor be under consideration for publication elsewhere (except conference proceedings papers). All manuscripts are thoroughly refereed through a single-blind peer-review process. A guide for authors and other relevant information for submission of manuscripts is available on the Instructions for Authors page. Applied Sciences is an international peer-reviewed open access semimonthly journal published by MDPI.
Please visit the Instructions for Authors page before submitting a manuscript. The Article Processing Charge (APC) for publication in this open access journal is 2400 CHF (Swiss Francs). Submitted papers should be well formatted and use good English. Authors may use MDPI's English editing service prior to publication or during author revisions.
Keywords
- Multimodal signal processing;
- Multimedia pattern recognition;
- Audio-visual fusion.