MaxEnt 2020/2021—The 40th International Workshop on Bayesian Inference and Maximum Entropy Methods in Science and Engineering
A special issue of Entropy (ISSN 1099-4300). This special issue belongs to the section "Multidisciplinary Applications".
Deadline for manuscript submissions: closed (20 December 2021) | Viewed by 20719
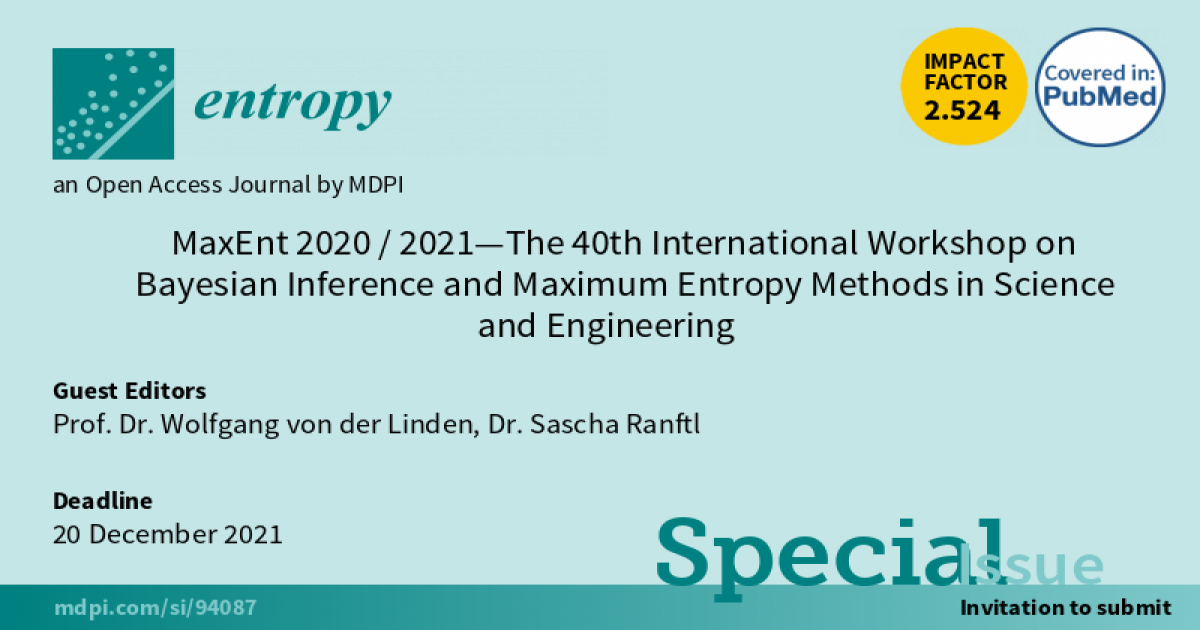
Special Issue Editors
Interests: Bayesian probability theory; stochastic processes; maximum entropy; condensed matter physics; quantum physics; quantum Monte Carlo; machine learning; neural networks
Interests: Bayesian probability theory stochastic processes; surrogate modelling; learning simulations; physics-informed machine learning; uncertainty quantification/uncertainty propagation of computer simulations; computational biomechanics; aortic dissection
Special Issue Information
Dear colleagues,
This Special Issue invites contributions on all aspects of probabilistic inference, such as foundations, novel methods, and novel applications. We welcome the submission of extended papers on contributions presented at the 40th International Workshop on Bayesian Inference and Maximum Entropy Methods in Science and Engineering (MaxEnt 2021). In light of the special circumstances and challenges of this year’s edition, we also welcome submissions that could not be presented at the workshop.
Contributions on foundations and methodology have previously addressed subjects such as approximate or variational inference, experimental design, and computational techniques such as MCMC, amongst other topics. Due to the broad applicability of Bayesian inference, previous editions have featured contributions to and from many diverse disciplines, such as physics (e.g., plasma physics, astrophysics, statistical mechanics, foundations of quantum mechanics), chemistry, geodesy, biology, medicine, econometrics, hydrology, image reconstruction, communication theory, computational engineering (e.g., uncertainty quantification), machine learning (e.g., Gaussian processes, neural networks), and, quite timely, epidemiology.
Prof. Dr. Wolfgang von der Linden
Dr. Sascha Ranftl
Guest Editors
Manuscript Submission Information
Manuscripts should be submitted online at www.mdpi.com by registering and logging in to this website. Once you are registered, click here to go to the submission form. Manuscripts can be submitted until the deadline. All submissions that pass pre-check are peer-reviewed. Accepted papers will be published continuously in the journal (as soon as accepted) and will be listed together on the special issue website. Research articles, review articles as well as short communications are invited. For planned papers, a title and short abstract (about 100 words) can be sent to the Editorial Office for announcement on this website.
Submitted manuscripts should not have been published previously, nor be under consideration for publication elsewhere (except conference proceedings papers). All manuscripts are thoroughly refereed through a single-blind peer-review process. A guide for authors and other relevant information for submission of manuscripts is available on the Instructions for Authors page. Entropy is an international peer-reviewed open access monthly journal published by MDPI.
Please visit the Instructions for Authors page before submitting a manuscript. The Article Processing Charge (APC) for publication in this open access journal is 2600 CHF (Swiss Francs). Submitted papers should be well formatted and use good English. Authors may use MDPI's English editing service prior to publication or during author revisions.