Topic Menu
► Topic MenuTopic Editors
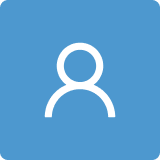
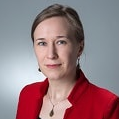
Methods for Data Labelling for Intelligent Systems
Topic Information
Dear Colleagues,
In our everyday life, we produce large quantities of data in different forms: sensor, video, audio textual data, etc. Labelling these data is a central part of the design and evaluation of intelligent systems that aim to understand and support the user. It is essential, both in designing and training a system, to recognize and reason about the situation and context, either through the design of new sensing modalities, the definition of suitable observation and semantic models in knowledge-driven applications, or though the preparation of training data for learning tasks in data-driven models. Hence, the quality of annotations can have a significant impact on the performance of the derived systems. Labelling is also vital for validating and quantifying the performance of intelligent applications, as well as for selecting the best performing setup of input modalities and configurations. Yet, high-quality annotations can have significant costs, often requiring significant time, expertise and funding, and so labelling tasks must be approached, with a pragmatic balance between quality, cost and use case in mind. With intelligent systems relying increasingly on large datasets with multiple heterogenous data sources, the process of data labelling is becoming a major concern for the community. To address the above challenges, this topic focuses on the following aspects of annotation: (1) Intelligent and interactive tools and automated methods for annotating large datasets, including the aspect of continuous learning and adaptation and life long learning, (2) The role and impact of annotations and annotations’ structures in designing intelligent systems, (3) The process of labelling, and the requirements to produce high-quality annotations, especially in the context of big data, (4) Methods for standardisation and normalisation in annotation practices. We invite you to submit works that offer new empirical or theoretical insights into the challenges and innovative solutions associated with data labelling, as well as on the impact that labelling choices have on the user and the developed system. The topics of interest include, but are not limited to:
- Methods and intelligent tools for annotating heterogenous data;
- Methods for standardisation and normalisation in annotation practices;
- Influence of interface on annotation;
- Processes of and best practices in annotating heterogenous data;
- Case studies in annotation for specific areas such as, but not limited to, wearable and ubiquitous computing, factory automation, medicine, healthcare, linguistics and law;
- Methods towards automation of the annotation process;
- Methods for improving and evaluating the quality of annotations;
- Ethical and privacy issues concerning data annotation;
- Beyond the labels: ontologies for semantic annotation of user data;
- High-quality and resuable annotation for publicly available datasets;
- Impact of annotation on a system’s performance;
- Building machine learning models that are capable of dealing with multiple (noisy) annotations and/or making use of taxonomies/ontologies;
- The potential value of incorporating modelling of the annotators into predictive models.
Dr. Emma Tonkin
Dr. Kristina Yordanova
Topic Editors
Keywords
- annotation
- labelling
- coding
- intelligent systems
- automated methods
- machine learning
Participating Journals
Journal Name | Impact Factor | CiteScore | Launched Year | First Decision (median) | APC |
---|---|---|---|---|---|
![]()
AI
|
- | - | 2020 | 20.8 Days | CHF 1600 |
![]()
Applied Sciences
|
2.7 | 4.5 | 2011 | 16.9 Days | CHF 2400 |
![]()
Data
|
2.6 | 4.6 | 2016 | 22 Days | CHF 1600 |
![]()
Sensors
|
3.9 | 6.8 | 2001 | 17 Days | CHF 2600 |
![]()
Systems
|
1.9 | 3.3 | 2013 | 16.8 Days | CHF 2400 |
MDPI Topics is cooperating with Preprints.org and has built a direct connection between MDPI journals and Preprints.org. Authors are encouraged to enjoy the benefits by posting a preprint at Preprints.org prior to publication:
- Immediately share your ideas ahead of publication and establish your research priority;
- Protect your idea from being stolen with this time-stamped preprint article;
- Enhance the exposure and impact of your research;
- Receive feedback from your peers in advance;
- Have it indexed in Web of Science (Preprint Citation Index), Google Scholar, Crossref, SHARE, PrePubMed, Scilit and Europe PMC.