Topic Menu
► Topic MenuTopic Editors



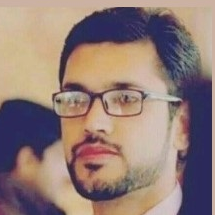

Advances, Innovations and Applications of UAV Technology for Remote Sensing
Topic Information
Dear Colleagues,
Nowadays, a variety of Unmanned Aerial Vehicles (UAVs) are commercially available and are widely used for several real-world tasks, such as environmental monitoring, construction site surveys, remote sensing data collection, vertical structure inspection, glaciology, smart agriculture, forestry, atmospheric research, disaster prevention, humanitarian observation, biological sensing, reef monitoring, fire monitoring, volcanic gas sampling, gap pipeline monitoring, hydrology, ecology, and archaeology. These less-invasive aerial robots support minimized user interventions, high-level autonomous functionalities, and can carry payloads for certain missions. UAV operations are highly effective in collecting valuable quantitative and qualitative data required to monitor isolated and distant regions. The integration of UAVs in such regions has substantially enhanced environmental monitoring by saving time, increasing precision, minimizing human footprints, increasing safety, and extending the study area of hard-to-access regions. Moreover, we have noticed a notable growth in emerging technologies such as artificial intelligence (AI), machine learning (ML), deep learning (DL), computer vision, the Internet-of-Things (IoT), laser scanning, sensing, oblique photogrammetry, aerial imaging, efficient perception, and 3D mapping, all of which assist UAVs in their multiple operations. These promising technologies can outperform human potentials in different sophisticated tasks such as medical image analysis, 3D mapping, aerial photography, and autonomous driving. Based on the aforementioned technologies, there exists an expanding interest to consider these cutting-edge technologies in order to enhance UAVs' level of autonomy and their other capabilities. Currently, we have also witnessed a tremendous growth in the use of UAVs for remotely sensing rural, urban, suburban, and remote regions. The extensive applicability and popularity of UAVs is not only strengthening the development of advanced UAV sensors, including RGB, LiDAR, laser scanners, thermal cameras, and hyperspectral and multispectral sensors, but it is also driving pragmatic and innovative problem-solving features and intelligent decision-making strategies in diverse domains. The integration of autonomous driving, collision avoidance, strong mobility for acquiring images at high temporal and spatial resolutions, environmental awareness, communication, precise control, dynamic data collection, 3D information acquisition, and intelligent algorithms also support UAV-based remote sensing technology for multiple applications. The growing advancements and innovations of UAVs as a remote sensing platform, as well as development in the miniaturization of instrumentation, have resulted in an expanding uptake of this technology in disciplines of remote sensing science.
This Topic aims to provide a novel and modern viewpoint on recent developments, novel patterns, and applications in the field. Our objective is to gather latest research contributions from both academicians and practitioners from diversified interests to fill the gap in the aforementioned research areas. We invite researchers to devote articles of high-quality scientific research to bridge the gap between theory, practice in design, and applications. We seek reviews, surveys, and original research articles on, but not limited to, the topics given below:
- Real-time AI in motion planning, trajectory planning and control, data gathering and analysis of UAVs;
- Image/LiDAR feature extraction;
- Processing algorithms for UAV-aided imagery datasets;
- Semantic/instance segmentation, classification, object detection and tracking with UAV data using the data mining, AI, ML, DL algorithms;
- Cooperative perception and mapping utilizing UAV swarms;
- UAV image/point clouds processing in power/oil/industry, hydraulics, agriculture, ecology, emergency response, and smart cities;
- UAV-borne hyperspectral remote sensing;
- Collaborative strategies and mechanisms between UAVs and other systems, such as hardware/software architectures including multi-agent systems, protocols, and strategies to work together;
- UAV onboard remote sensing data storage, transmission, and retrieval;
- Advances in the applications of UAVs in archeology, precision agriculture, yield protection, atmospheric research, area management, photogrammetry, 3D modeling, object reconstruction, Earth observation, climate change, sensing and imaging for coastal and environment monitoring, construction, mining, pollution monitoring, target tracking, humanitarian localization, security and surveillance, and ecological applications;
- Use of optical laser, hyperspectral, multi-spectral, and SAR technologies for UAV-based remote sensing.
Dr. Syed Agha Hassnain Mohsan
Prof. Dr. Pascal Lorenz
Dr. Khaled Rabie
Dr. Muhammad Asghar Khan
Dr. Muhammad Shafiq
Topic Editors
Keywords
- drones
- UAVs
- aerial robots
- remote sensing
- aerial imagery
- LiDAR
- machine learning
- atmospheric research
- sensing and Imaging
- processing algorithms
Participating Journals
Journal Name | Impact Factor | CiteScore | Launched Year | First Decision (median) | APC |
---|---|---|---|---|---|
![]()
AI
|
- | - | 2020 | 20.8 Days | CHF 1600 |
![]()
Drones
|
4.8 | 6.1 | 2017 | 17.9 Days | CHF 2600 |
![]()
Inventions
|
3.4 | 5.4 | 2016 | 17.4 Days | CHF 1800 |
![]()
Machine Learning and Knowledge Extraction
|
3.9 | 8.5 | 2019 | 19.9 Days | CHF 1800 |
![]()
Remote Sensing
|
5.0 | 7.9 | 2009 | 23 Days | CHF 2700 |
![]()
Sensors
|
3.9 | 6.8 | 2001 | 17 Days | CHF 2600 |
MDPI Topics is cooperating with Preprints.org and has built a direct connection between MDPI journals and Preprints.org. Authors are encouraged to enjoy the benefits by posting a preprint at Preprints.org prior to publication:
- Immediately share your ideas ahead of publication and establish your research priority;
- Protect your idea from being stolen with this time-stamped preprint article;
- Enhance the exposure and impact of your research;
- Receive feedback from your peers in advance;
- Have it indexed in Web of Science (Preprint Citation Index), Google Scholar, Crossref, SHARE, PrePubMed, Scilit and Europe PMC.